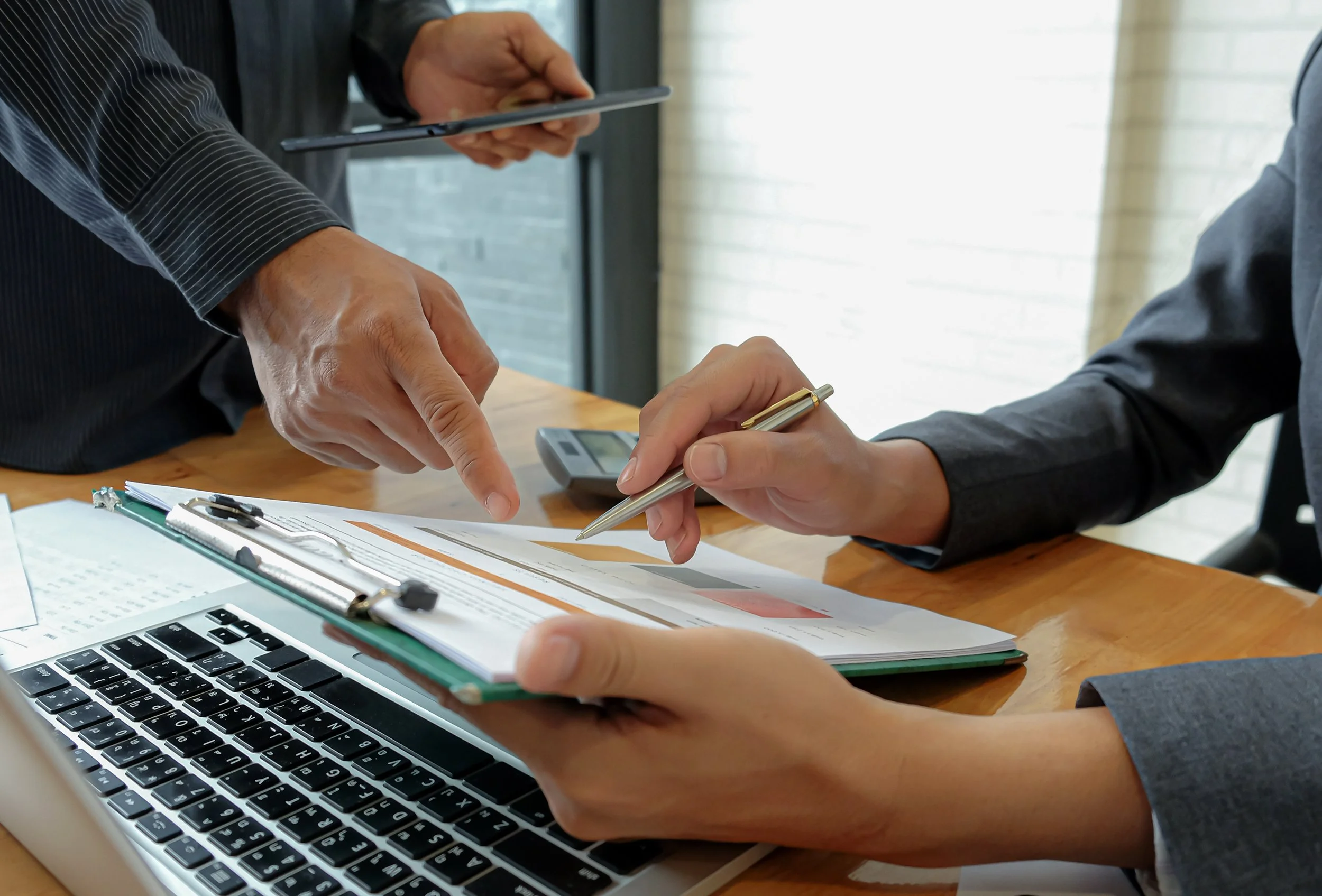
Monte Carlo Simulation
Because the future is both uncertain and unknowable, investment planning frequently involves efforts to simulate or “model” the future. Given certain investment decisions, how likely is it that a particular portfolio will succeed in reaching the goals set for it? For example, will a portfolio provide enough retirement to last the owner’s lifetime? Will the expected investment results pay for a child’s education?
One way to analyze such questions is through use of a “Monte Carlo” simulation.
What is a Monte Carlo Simulation?
From a theoretical perspective, a Mont Carlo¹ simulation is a method of estimating the probable outcome of an event in which one or more of the variables affecting the outcome are chosen randomly. The essence of the Monte Carlo process is to simulate a process or event many times. During each simulation, the variables which affect the outcome are allowed to fluctuate according to pre-selected criteria. The outcome of each simulation is then ranked according to the likelihood of its occurrence.
As a very simple example, how often would an individual player win at the card game Solitaire? The Monte Carlo approach would have the individual play 100 (or even more) games of Solitaire and then record the results. The number of successful plays is the “probability” of winning the game.
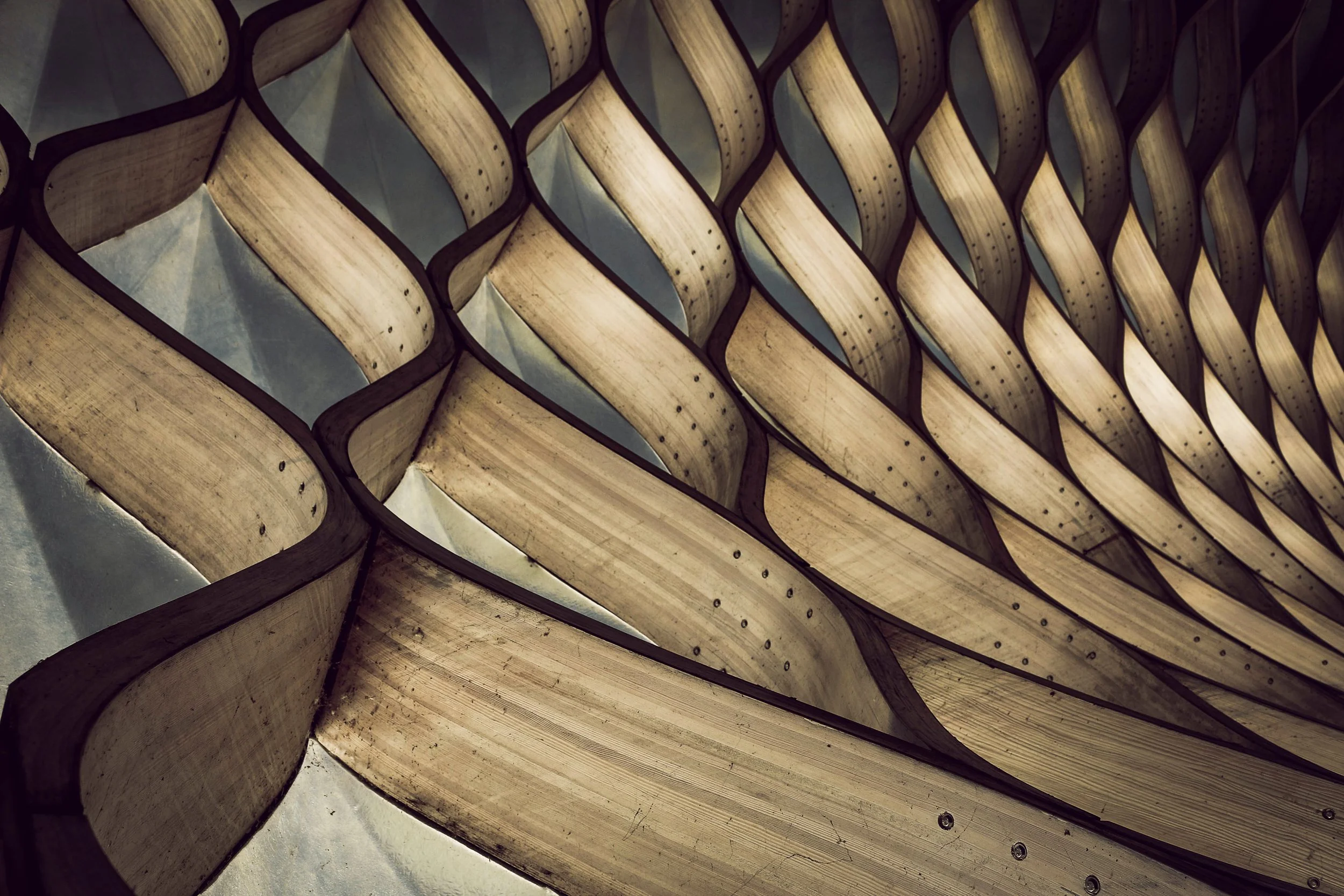
Monte Carlo Simulation and
the Investment World
In the investment world, a Monte Carlo simulation is useful in helping an individual investor understand the role that risk plays in portfolio selection and design. Investing does involve risk, including the possible loss of principal.
For example, rates of return for stocks, bonds, and other investments will vary from year to year. Depending on the historical time frame chosen, a particular investment will have good years and bad years. From this historical data, it is possible to calculate highs, lows, and average rates of return for the investment. The analysis will also determine how widely the annual rates of return varied from the average, resulting in a distribution of probable rates of return around the average.
To estimate the return that a particular investment or portfolio could achieve over a future time period, a Monte Carlo simulation generates a rate of return for each year in the analysis, based on the historical distribution of probable rates of return. In more complex simulations, economic data such as inflation, withdrawals and contributions, and income tax rates are also included. This process is repeated numerous times until the simulation is then ranked by percentiles. In the case of a retirement analysis, “success” would be measured by having enough money to last until the expected mortality. Failure would be running out of money early.
A Monte Carlo simulation is useful in portfolio design in that multiple scenarios can be simulated, with varying portfolio mixes.
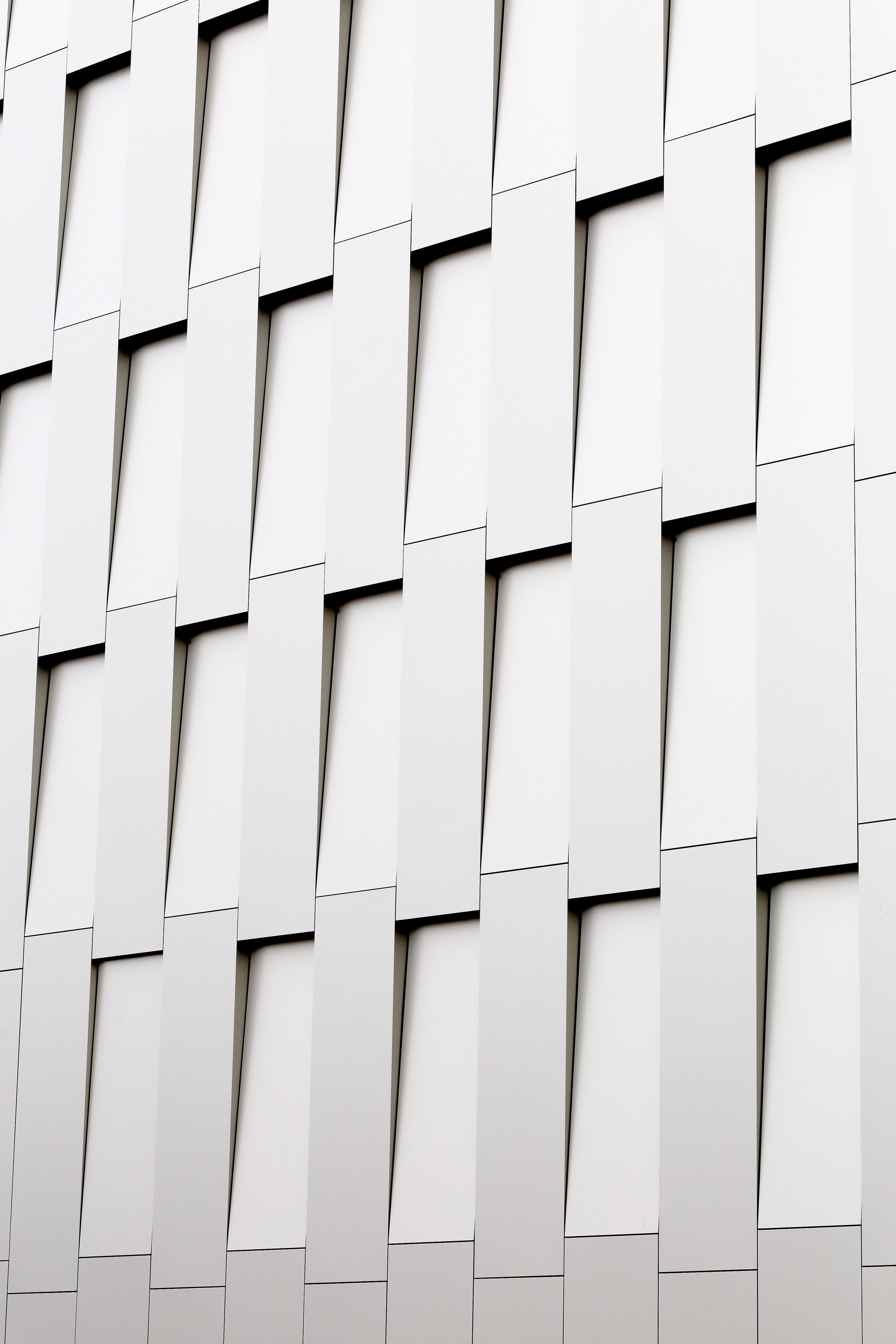
What does a Monte Carlo
Simulation look like?
The graph shows the results of a typical monte Carlo simulation:
Graph Source: Advisys
Limitations of the Monte Carlo Simulation methodology
All hypothetical projections of future investment results, of whatever type, should be regarded as only approximations or a “best guess” as to what the future might bring. Although a carefully constructed Monte Carlo simulation can shed light on the potential risks and rewards of a particular investment or portfolio, it should not be regarded as predicative or a guarantee of future results.
Seek Professional Guidance
When addressing any investment or planning question, the guidance of trained, experienced financial professionals is strongly recommended.
Source: Advisys, Inc. 2022